In our last blog post, we explored the limitations of physics-based models for camera perception. End-to-end deep learning models are powerful, but its complex structure means the decision making process is near impossible to understand. With far fewer parameters and rigorous assumptions about how objects can move in the external world, physics-based models are interpretable, but lack the complexity needed to accurately predict human behaviour in all scenarios. It’s clear automated systems are missing something when it comes to crossing prediction.
March 17, 2021
At Humanising Autonomy, we believe true understanding of pedestrians’ cognitive processes makes all the difference. A driver knows if a pedestrian is aware of happenings on the road, just as a pedestrian knows whether it’s safe to cross. This innate understanding of human behaviours and intentions, or theory of mind, is essential for the driver in making appropriate decisions and helps to identify when further communication is required. Moreover, this perspective is necessary to bridge the critical safety gap in the industry’s current approach to prediction models.
The theory behind our behavioural models
It’s clear that the driver intuitively knows what the pedestrians’ intentions are and how to respond. This unspoken language between people on the road and drivers is difficult to translate into predictive models, but not impossible. We invest a great deal of behavioural research into understanding how we can infer the underlying cognitive processes from contextual and dynamic information of the scene and how those can be combined within probabilistic machine learning models to predict pedestrian crossing accurately. We called this the Behavioural Model approach.
To understand the contribution of our Behavioural Model, we conducted a series of studies that compared industry-accepted physics models against our model on crossing prediction performance. The data from one such study is shown below.
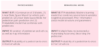
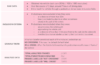
What’s the prognosis?
Overall, the results highlight the limitations of physics models, and how these could be overcome with our Behavioural Model.
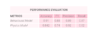
The models were compared frame-by-frame in terms of their prediction performance (see Figure 1). Here, the F1 score is the most informative metric as it combines precision and recall to account for unbalanced datasets. Based on the F1 score, the Behavioural Model reduced the error of physics models by 53%. Visual inspections of the predictions showed that the much lower recall of the physics model was mainly caused by a delayed crossing prediction.
Meanwhile, the slightly lower precision of the Behavioural Model was mainly caused by input feature noise. We are continuously improving the input features to reduce the risk of false positives.
Next, we analysed the different performance metrics over time. Results revealed that the Behavioural Model outperformed the physics model at any time and predicted crossing with high accuracy up to 4 seconds in advance; gradually increasing for shorter predictions (see Figure 2).
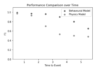
Note that all metrics would be higher when including all pedestrians (we excluded simple cases), and that the prediction performance is reported relative to an attentive human labeller who could annotate each individual pedestrian frame-by-frame. This can be considered ‘superhuman performance’ and is certainly superior to any driver prediction performance in real-time.
These results demonstrate that, without capturing the underlying psychological processes of pedestrian behaviour, physics models fail to accurately predict pedestrian crossing. Predictions can be wrong or delayed, which makes driving through downtown more difficult and potentially dangerous. By incorporating psychology into probabilistic machine learning models, Humanising Autonomy is able to mitigate the limitations of physics-based models while keeping the positive attributes of a white box approach: interpretability, transparency, small model size and a trustworthy estimate of its prediction uncertainty.
This is the second in a series of blog posts by Senior Behavioural Data Scientist Dominic Noy. His webinar Beyond Physics: Tackling the Limitations of Camera Perception is available now for download. Contact [email protected] to learn more.